AI Inclusivity and Design Thinking
Add bookmark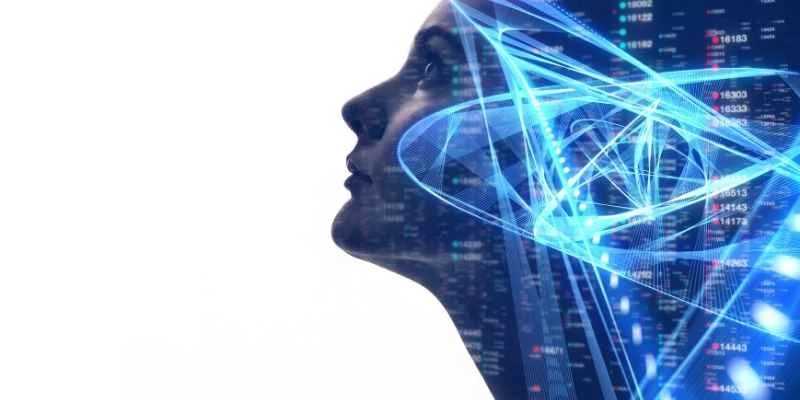
Artificial intelligence is continuing to become more prevalent within the job hiring and recruitment process. These technology-driven tools are utilized to sift through immense numbers of job applications in short periods of time, evaluate responses to written questions, and essentially eliminate any candidates considered unfit for a given position.
There is some clear hesitation associated with this process, and, if not considered in a more thoughtful and fair way, this approach can lead to some serious human biases that may have long-term negative effects on the future of the hiring process. Identifying these biases early on is the only way to properly resolve such prejudices from being unchecked and becoming further entrenched.
Biased AI Algorithms
AI programs and algorithms are only as neutral and fair as their programmers, designers, and the data they have been trained on. If you design a hiring model to identify with your current workforce demographics, which may not be necessarily diverse, then the machine will learn accordingly, and potentially learn to favor such discriminating characteristics.
Bias is a human flaw, not a technological one. These machines are being trained to read and talk through data sets inclusive of specific words and phrases. The resulting data, if not looked at in great detail, can reflect the biases of their designers, and inherently evolve into an algorithm based not on inclusivity, but on an uneven playing field.
New research has shown that there are subtle gender biases entrenched within the very data sets that have been training language skills and algorithms to these AI hiring programs. It is important to recognize this and act quickly, so designers can actively consider their own biases and use more impartial data going forward.
"Feminist Design Thinking"
Researchers from North Carolina State University and Pennsylvania State University have studied these well-established biases, and developed the concept of “feminist design thinking” in order to act on these issues and improve gender equality, especially when considering the hiring process.
If design thinking guidelines and strategies are implemented into the development process, these AI systems can be more meaningful and inclusive to all individuals, including those who currently may be under-represented but just as qualified for a given position.
According to researcher and professor Fay Payton, “Too many existing algorithms incorporate de facto identity markers that excluded qualified candidates because of their gender, race, ethnicity, age and so on…We are simply looking for equity – that job candidates be able to participate in the hiring process on an equal footing.”
How does it work?
Taking this feminist design thinking approach means being mindful of potentially biased data and incorporating this idea of equity directly into the design of these hiring algorithms. Design thinking can serve as an effective way to think more mindfully about inclusion and human-centeredness when developing these systems.
According to Payton, “this approach would mean developing algorithms that value inclusion and equity across gender, race, and ethnicity…Essentially, developers of all backgrounds would be called on to actively consider – and value – people who are different from themselves.”
This goes beyond just doing what is ethical. It’s no news that companies boasting a more diverse workforce, particularly in executive and leadership roles, see higher profits and longer-term value creation. McKinsey & Company’s most recent report on diversity and financial success has proven the connection between the two is evident. McKinsey found that in 2014, “companies in the top quartile for gender diversity on their executive teams were 15 percent more likely to experience above-average profitability than companies in the fourth quartile,” and within their expanded 2017 data set, “this number rose to 21 percent and continued to be statistically significant.” In addition to this, they found that top-quartile companies in this regard “had a 27 percent likelihood of outperforming fourth-quartile peers on longer-term value creation."
Overall, as AI programs continue to permeate the recruitment and hiring processes, it's important to be mindful in the design and implementation of these systems in order to achieve the most moral – and profitable – results.